So, in the realm of ones and zeros spun, ChatGPT emerged, a dialogue begun. In the digital tapestry, its story sown, A testament to the code, a creation well-known.
ChatGPT

ChatGPT and other Large Language Models have come to the forefront of AI research and public discourse over the last couple of years. What is this AI technology and where will it take us? I will discuss this in my next three blogs. First: what is the technology behind ChatGPT?
ChatGPT and its brethren, together called Large Language Models, are the latest in a long line of artificial neural networks, one of the approaches to AI that has been pursued since the 1950s. The artificial neural network approach to AI was inspired by the discovery that the human brain and other biological nervous systems are large networks of interconnected cells, neurons, each of which fires an output signal if its input signals together exceed a threshold.
This led AI researchers to the idea that large networks of simple computational units might lead to smart systems. An example of such a simple computational unit is shown below:

This computational unit (also called an “artificial neuron”) takes inputs from four other units, multiplies each input by a weight, adds up the inputs multiplied by the weights, and compares this sum to a threshold. If the sum is greater than the threshold, the unit outputs a value of “1”, otherwise the unit outputs “0”. This output is sent to other units in the network.
How the unit responds to its inputs is controlled by the values of the weights W and the threshold T. The weights and the threshold are referred to as the unit’s parameters.
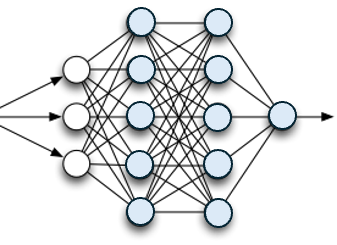
Inspired by neural networks in the brain, these simple computational units can be put together into artificial neural networks like the simple one on the left.
Early work with artificial neural networks demonstrated that they could indeed recognize simple patterns, e.g., handwritten text or spoken words. Even more exciting, mathematical techniques were developed to automatically adjust the parameters of the computational units so they gave correct answers, using just examples of inputs and correct outputs. This was the beginning of machine learning, the process by which artificial neural networks are “trained” using training samples.
These early demonstrations of “electronic brains” systems that could “learn” from examples got people pretty excited. The term “artificial intelligence” was coined and people began worrying about robot overlords. Note, this was in the 1950s!
As early research progressed, it became clear that these artificial neural networks were quite limited in what they can do. Tasks such as recognizing objects in pictures and translating language seemed just too complicated for artificial neural networks. AI research went in new directions to try to capture the complexities of human intelligence and the world it inhabits.
As the decades marched on, a few die-hard researchers continued to explore artificial neural networks. Finally, about 10 years ago, they had their day. Improvements in computer technology and the availability of large amounts of training data on the internet allowed very large artificial neural networks to be built and trained. It was discovered that these “deep neural networks” performed very well, better than anyone expected.
ChatGPT is an example of one of the most recent developments in deep neural networks. It is huge! Recall that an artificial neural network’s calculations are controlled by parameters that are set through the training process using training samples. The simple network above has 56 parameters. The latest version of ChatGPT has about 100 trillion parameters!
Besides its size, ChatGPT has three distinctive features indicated by the “GPT” part of its name:
- It is Generative – it generates text that completes missing parts of the text that is given as its input
- It is Pre-trained – its parameters were adjusted by having it predict removed portions of text in a training set that included about 300 billion words
- It is based on the Transformer architecture, which is a particular way of connecting the computational units in its deep neural network.
ChatGPT and other Large Language Models have demonstrated impressive capabilities. They can create news stories, essays, poetry, legal documents, translations, summarizations, computer code, and other documents. These tools are expected to transform businesses and other aspects of our society. In future blogs we will explore these impacts, including the risks and dangers of this technology.